Seven Ways Engineers Use Data Analytics
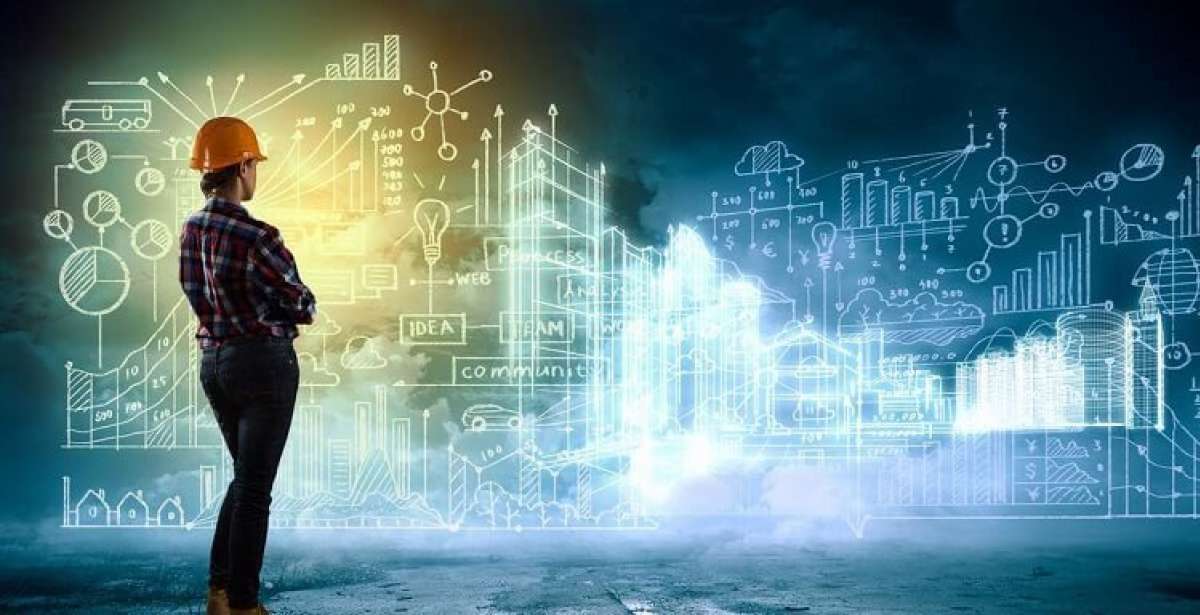
As technology evolves at an ever-increasing rate, engineers across a host of industries must stay on top of new developments and information. In a paper entitled “Big Data: A Survey Research Perspective” shared during the Statistics Canada Symposium 2016, author Reg Baker defined Big Data as “a term that describes datasets so large and complex that they cannot be processed or analyzed with conventional software systems.” Big Data continues to become an integral part of modern work—one that engineers who have their sights set on leadership in their fields will benefit greatly from understanding.
Such a landscape requires professionals eager to be lifelong learners. “Data engineering is a job that takes a lifetime to master," Stephanie Tam, an engineering director for Kyruus, which has leveraged data to become the industry leader in provider search and scheduling solutions for health systems, told Stitch Data. "Every year there's something new to learn. You're never doing the same thing year after year."
An online Master of Engineering Management (MEM) degree can offer engineers the chance to enhance their career possibilities by learning about and leveraging data analytics engineering. Students graduate equipped to use data analytics applications to effectively lead teams within technological fields. Graduates are ready to mobilize money, people and technology to analyze, innovate, optimize and excel in the engineering field using engineering data analysis systems that help them assess risk and streamline processes.
In this post, we’ll share seven ways that engineering managers utilize data.
Learn More About uOttawa's Online MEM Program
Streamlining Processes through Data Analytics Applications
Big Data analytics not only provide massive sets of information, but provide analysts with the opportunity to make future predictions based on trends in the data sets. For example, “Big Data analytics can give manufacturers more lead time, providing the opportunity to produce customized products almost as efficiently as goods produced at greater scale. Innovative capabilities include tools that allow product engineers to gather, analyze and visualize customer feedback in near-real time.”
In addition to providing pathways for customer feedback to be communicated quickly and clearly, engineering data analysis can identify bottlenecks and supply chain problems, which enables engineers to make adjustments that increase efficiency. Complex models that are built from real — and real-time — data “don’t make unrealistic assumptions about productivity. And they automatically incorporate the effects of the everyday delays and disruptions that development teams must face. In other words, they take into account not only the complexity of the project (both the functional and implementation aspects) but also the complexity of the team environment.”
With this information at their disposal, engineering managers can better streamline their processes and empower their teams to work effectively and productively.
Blog: Business Intelligence Tools for Engineers
Team Leadership
In their book Managerial Analytics: An Applied Guide to Principles, Methods, Tools, and Best Practices, authors Michael Watson, Peter Cacioppi, and Derek Nelson define managerial analytics as “what a manager needs to know about the field of analytics to make better decisions.” For managers, data analytics can provide crucial information that informs the way they lead their teams, assign projects, and make hiring decisions.
Data analytics can provide engineering managers with critical information about the employees in their companies or organizations. This type of analysis is often called People Analytics or Relational Analytics. It’s designed to provide statistical information that informs hiring and team management decisions.
For example, an American automobile corporation leveraged people analytics to form collaborative teams. “We are very careful about who we select,” said the program leader. “We get the people with the right functional backgrounds, who have consistently done innovative work, and we make sure there is a mix of them from different backgrounds and that they are different ages.”
LinkedIn’s 2020 Talent Trends report stated that 73% of talent professionals say their organizations see people analytics as a “major priority” as they look toward the next five years. And yet, over half of them are yet to have a sufficient data analytics team ready to uphold that priority. This is a tremendous opportunity for skilled data analytics engineering professionals who have a strong understanding of data analytics applications as well as management skills.
Risk Assessment and Management
In “The Role of Risk Assessment in Engineering Practice,” Mobayode Olusola Akinsolu defines risk assessment as “the determination of the measure and value of risk related to an existing situation and a known threat.” Engineering managers play a critical role in assessing and mitigating the risks adjacent to the projects they oversee.
According to Nate Richards, President of Entrance Software Consulting, engineering firms can also use data for risk assessment by “monitoring sensor data for structural integrity.” He writes, “Most successful industrial manufacturing companies are already using sensors for exception monitoring to prevent unexpected structural problems. Imagine if a bridge, building, or road could send you an alert when it needs your immediate attention. The applications and usage scenarios here are endless.”
Cihan Biyikoglu, Executive Vice President of Product at RMS, “the world's leading provider of products, services, and expertise for the quantification and management of catastrophe risk,” says that the Risk Data Open Standard (RDOS) will better integrate data “between systems that deal with exposure and loss data” which will help leaders like engineering managers to “better understand the type of protection we need to create against climate change and other natural or man-made disasters.”
Producing Dashboards and Reports
In a post entitled “Analytics for the Engineering Manager,” Engineering Manager at Pager Duty Leeor Engel writes about several ways he uses data analytics applications in the reports he produces. Examples include:
- Using JIRA and an ETL tool to create project forecasting reports. Engel writes that he uses these reports directly with the engineers on his team so that they can track progress, “make course corrections,” and have a shared “live updated project scoreboard”
- Leveraging tools like PeriscopeData, Mode, and Tableau to facilitate spaces conducive to querying data and producing dashboards
- Producing ad-hoc reports that clearly present data trends and patterns to stakeholders
Managers will also find data visualization tools—applications that allow them to interact with aggregated data in appealing, useful ways—to enhance their strategic and operational management capabilities. Stephanie Ray at Project Manager lists three ways that data visualization can help managers:
- Informational Graphics: Many engineering projects require a tremendous amount of complicated data to be synthesized and comprehended quickly. Informational graphics, Ray says, “help people see patterns in the data, such as trends that might not be as obvious without the visuals.
- Visual Literacy: Some people process and retain information more effectively through pictures rather than words. “Data is communicated and understood by a viewer’s ability to interpret meaning from the image,” Ray says. “Literacy is not solely regulated to words.”
- Exploratory Data Analysis: “By modeling data sets in a visual context, main characteristics can be highlighted and summarized,” says Ray. This fits well with the ways that data analysis and visualization can interact with business intelligence tools—analysis and visualization empower managers and their teams to interact with data in visual, comprehensible ways, and business intelligence tools can transform that analysis into reports that inform business decisions.
Engineering managers can use data analytics applications to advocate for their teams. Squarespace Engineering Manager Aldo Garcia told Built In that his job includes managing “four teams that build internal applications and data pipelines to help Squarespace be more efficient and data-informed.” For Garcia, collecting data includes getting to know every team member who reports to him so that he can “understand what motivates them and help them grow by providing tailored feedback.” Garcia also works with the leadership team at Squarespace to incorporate the in-house employee data he’s analyzing in order to achieve goals on both the project and institutional culture sides of the business.
Measuring Productivity and Performance Management
Andy Montgomery, senior vice president of engineering at Aurea, says that after just a month of using a data program that analyzes performance data, he “projected a 50% increase in productivity and a 20% reduction in the team’s operating costs by the end of the quarter.”
Similarly, Vicki Speed at Inside Unmanned Systems magazine reported that “Calgary, Canada-based Integrated Sustainability, a sustainable infrastructure delivery and operations company, is seeing visible improvement with the use of machine control and, more recently, autonomous systems. By leveraging data, crews are increasing production in the neighborhood of 30 to 45 percent.”
These are exactly the types of problems that engineering manages who know how to leverage data analytics applications can solve. By building pipelines and models that make data readily available to fellow workers who need it, data engineers and engineering managers find quantitative gaps in productivity and configure solutions to eliminate them.
Determining Success
Dr. Nicole Forsgren, co-founder, CEO and Chief Scientist at DevOps Research and Assessment (DORA), says managers should “use measures that focus on outcomes, not output,” and “use measures that optimize for global or team outcomes, not local or or individual outcomes.” Take a software engineering manager, for example. Forsgren says that, rather than determining success based on lines of code written in a certain amount of time, the manager should focus on measurements like “tempo, stability, database, and quality.”
By collecting data like:
- How long it takes a team to deploy code
- Change fail rate
- Gaps between database and development teams
- How much time is spent on patches
Software engineering managers can determine what success could look like for their teams, then take the steps to help them achieve it.
Similarly, data analytics applications can provide critical information to construction and civil engineering managers as they set out to define and determine success for their teams and projects. According to Ryan Ayers at Dataconomy, “Big data analytics can deliver reliable financial data, specific event alerts and complex analytics, all in real time. The technology allows civil engineers to verify and analyze information amazingly fast and avoid project pitfalls that can make the difference between success and failure.”
Engineers may also find data analytics helpful in establishing and measuring key performance indicators (KPIs), “a metric of performance and determines if you are achieving success in an organization.” Construction Dive quoted, Jit Kee Chin, executive vice president and chief data officer at Suffolk Construction, who said, “What you haven’t measured, you can’t manage.” She later added, “Prospective clients will come to expect access to KPI-based performance data and use that information to make hiring and contract decisions.”
With meaningful analytics at their fingertips, engineering managers can clearly define success, realistically assess the barriers in their way, and form strategies that render success achievable.
Improving Products
Ann Peedikayil worked at Caterpillar for over a decade in various engineering roles before transitioning into a data science role in 2018. During her engineering roles, Peedikayil said in an interview, she “used data to help develop solutions for continuous product improvement (CPI) and helped direct new product introduction (NPI) design decisions.”
Peedikayil sees data analytics not simply as an investigation into interesting information, but as a true end-to-end asset that benefits every level of production. “Data analytics has helped me to understand our customers, our dealers, our machines and our processes at various levels of granularity,” she said.
According to an article entitled “Big Data in Design and Manufacturing Engineering” by Lidong Wang and Cheryl Alexander in the American Journal of Engineering and Applied Sciences, “Some manufacturing firms, such as General Electric, view Big Data from sensors in manufactured products (e.g., locomotives, jet engines and gas turbines in GE’s case) as key to effective and efficient servicing strategies. In the same mode, automobile manufacturers such as General Motors created self-driving cars based on the analysis of Big Data from sensors and machine vision technologies (Davenport, 2013). Big data has become the key asset for the whole production and manufacturing cycle, as well as the provision of services in the automotive and mobility space. Big Data is actually at heart of how the extracted sensor data and location data are combined to provide services (Camilli and Duisberg, 2013).”
Big Data, when analyzed and understood, directly correlates to product improvement.
Incorporating AI
Cognilytica found that “Data preparation and engineering tasks represent over 80% of the time consumed in most AI and Machine Learning projects.” This fact alone makes it clear—in a world with an increasing focus on AI, managers with data analytics engineering skills are in demand. Data engineers collect and store data for further usage, so they may not build artificial intelligence themselves, but the building can’t happen without the data they provide. Machine learning engineers, on the other hand, “sit at the intersection of software engineering and data science...machine learning engineers also build programs that control computers and robots.”
Chemical engineers use data analytics in order to incorporate artificial intelligence into their processes and industries, as well. Henkel AG & Co. KGaA, for example, launched the Smart Operations initiative, which collects internal and customer data related to Henkel products. The initiative has led to “many AI benefits, from expediting speed to market for new product formulations and scaleup to rapidly detecting and resolving product-quality issues.”
As for civil engineers, “when big data technology produces solutions that humans cannot readily identify, AI will step in to make sure that savvy civil engineers don’t miss out on an opportunity for improvement.” Rather than big data, data analytics, or artificial intelligence simply becoming one more overwhelming task on the to-do list of an already-busy engineering manager, they can be leveraged to interact in ways that aid engineers in adapting to new technologies while achieving their project goals.
Engineers in manufacturing and production line management will find that Smart Factory, “a highly digitalized and connected environment where machinery and equipment are able to improve processes through automation and self-optimization, leverages data in such a way that connects “the top floor to the shop floor.” Artificial Intelligence can also detect device failures or defects and test new designs. Additional uses of artificial intelligence for manufacturing and product engineers include Artificial Intelligence Decision Support Systems (AI-DSS), AI-based process optimization, Artificial Intelligence/Machine Learning-based defect detection, Artificial Intelligence/Machine Learning-based maintenance prediction and Industry 4.0 digital automation processes.
Becoming a Data Analytics Engineer and Leader
If you want to become a leader in your field, the online Master of Engineering Management (MEM) program at the University of Ottawa can help you reach your goal.
The MEM program:
- Teaches essential management and leadership skills
- Features industry-expert faculty and accomplished alumni
- Promises flexibility, concision, a part-time pace, and a fully online experience
Our data analytics degree module teaches powerful analytics techniques to help professionals communicate data insights to support their decision-making. Whether you’re a structural engineer who desires to leverage data for predicting impacts, a software developer who wants to understand more thoroughly how data can improve project outcomes, an aspiring engineering manager with your sights set on excellent data presentation, or represent one of a host of other engineering roles, the skills you gain during the MEM program will equip you to advance your career.
Learn more about uOttawa’s online Master of Engineering Management degree - Data Analytics module.
Read more of uOttawa online's top blogs below:
1. Engineering Management: the Year in Review
2. Engineering Management: The Complete Guide to Leading Technical Teams
3. Engineering Management Master’s vs MBA: What's the Difference?